Effective Strategies to Mitigate Cybersecurity Risks to AI
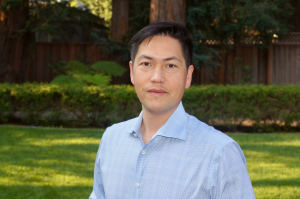
As artificial intelligence (AI) becomes increasingly woven into business operations, security teams face a complex challenge: developing scalable risk management strategies that address the nuances of this emerging technology.
This challenge is particularly pronounced when focused on the risks inherent in AI model development and usage, especially as they span complex environments such as on-premises systems, cloud infrastructure, or hybrid networks. In particular, innovations like Retrieval-Augmented Generation (RAG) allow even small LLMs to outperform the largest LLMs by allowing them to reference documents as they generate responses. And as AI becomes more embedded in corporate workflows, customers need continuity and reliability of access to it.
As a result, security teams need cybersecurity solutions that can deliver dynamic and robust security to protect the proprietary data and sensitive access often granted to AI without compromising productivity or limiting scalability.
Let’s dive into this challenge and explore three proven strategies to find this security sweet spot:
The Need for Scalable AI Risk Mitigation
The types of problems AI is being applied to are staggering - ranging from simple productivity enhancements for routine tasks, all the way to advanced chip development.
The common element in each of these applications is the need for AI models to have access to powerful processing capabilities, data sources, and networking appliances that enable their use cases.
However, the weaknesses of traditional cybersecurity solutions are often amplified when charged to mitigate the unique challenges posed by AI systems. These limitations stem from several factors:
- Adversaries: Sophisticated attackers are increasingly targeting AI systems, recognizing their potential access to proprietary data and enabling applications and datasets.
- Platform limitations: Traditional cybersecurity solutions frequently struggle to apply data controls dynamically across multiple platforms and environments (cloud, on-premises, hybrid.)
- Continuous Availability: Cybersecurity solutions often fail to maintain uninterrupted access to AI systems and data, which a lead to potential downtime and loss of productivity.
In order to effectively mitigate risks associated with AI, organizations need cybersecurity solutions that can adapt to the dynamic nature of AI systems. This requires a shift towards more advanced and flexible security architectures.
3 Proven Risk Mitigation Strategies to Strengthen Resilience with AI
Zero Trust Architecture
One of the most effective strategies for mitigating AI-related risks is implementing a Zero Trust architecture. This approach creates secure virtual chambers around applications by using packet-level inspection to validate all users, devices, and software clients. This validation happens at every interaction without slowing down network traffic or inhibiting AI querying.
By implementing a Zero Trust architecture, organizations can layer defenses around the AI cluster, preventing unauthorized access to or from it. Zero Trust can then expand, leveraging policies and lessons learned, to protect the source documents that AI needs to access.
This approach significantly reduces the risk of unauthorized access to AI systems and their associated data while also offering a dramatic reduction of the attack surface of AI systems.
Identity and Access Management
Another proven strategy for AI risk mitigation complements Zero Trust: robust Identity and Access Management (IAM).
Implementing IAM involves implementing tools that enable and enforce:
- Least privilege access: Granting users (and AI agents!) only the minimum necessary permissions for their roles.
- Multi-factor authentication: Adding an extra layer of security beyond simple passwords, such as token or biometric authentication, for enhanced verification
- Account management: User accesses and permissions are controlled across a network environment. Account management can be integrated with other systems, such as human resources, to automatically update account information.
Properly implementing IAM ensures that only authorized personnel can interact with AI systems while also limiting the potential damage from compromised credentials.
Continuous Monitoring and Threat DetectionFinally, security teams can turn toward advanced threat detection to help maintain the security of AI-related systems.
For example, machine learning algorithms can be used to analyze large amounts of data in real-time, identifying anomalies and tactics, techniques, and procedures (TTPs) of potential attacks. Similarly, integration with Security Information and Event Management (SIEM) systems enables quick incident response to emerging threats, which allows organizations to detect and respond to threats before they escalate into full-blown attacks.
Creating a Resilient Network
Scaling risk mitigation as AI usage expands isn't just about deploying more tools. instead, it requires a comprehensive approach that integrates advanced strategies, continuous monitoring, and resilient architectures to ensure AI systems remain secure as they expand in use.
While the future of AI and its potential is just beginning to be understood, the core principles outlined here—Zero Trust architecture, robust IAM, and continuous monitoring—provide a solid foundation for building a secure AI ecosystem.
Ultimately, the goal of AI security is not to completely eliminate risks but to create a resilient environment where the benefits of AI can be realized safely and efficiently. By adopting these strategies, organizations can build trust in their AI systems and unlock their full potential for innovation and growth.