AI and Cybersecurity Infrastructure Risks and Shortcomings
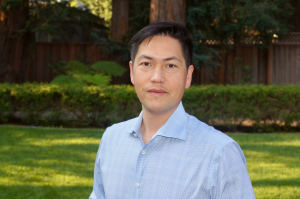
Once a technology reserved for select research labs, artificial intelligence (AI) has quickly become integral to many businesses' operations.
Although this rapid integration has brought with it many exciting opportunities and advantages, the cybersecurity community is just now beginning to understand the full scope of the risks the technology can bring to their networks.
The stakes only get higher with the integration of large language models (LLMs), which leverage deep learning to understand vast internal and external datasets and generate content, offering unprecedented business applications and capabilities in natural language processing (NLP).
Fortunately, security professionals have begun to use several key best practices and platforms to mitigate the risks and challenges associated with AI. This article explores those risks and how your organization can maximize AI and LLM potential without sacrificing security and privacy.
LLMs: What You Need to Know
LLMs represent a significant advancement in AI, leveraging deep learning techniques to understand extensive datasets, process requests, and generate content. Because of how they are tuned, these models are particularly adept at natural language processing (NLP), enabling users to provide prompts in natural language and receive relevant information back.
The life of an LLM begins with a multi-phase training process that includes exposure to unstructured and unlabeled data. This data teaches the model to identify relationships between words and concepts. Over time, this foundational training is enhanced by sessions with labeled data, refining the model's accuracy and precision.
Deep learning plays a pivotal role in the functionality of LLMs. It involves the use of neural networks with several layers (hence the term "deep") that enable the model to perform complex pattern recognition and feature extraction tasks through a mechanism known as self-attention. Self-attention allows the model to weigh the relevance of each word in relation to every other word in a sentence or document. This capability means it can capture more intricate dependencies and nuances in the language to mimic humanlike understanding and language generation.
How an LLM Effectively Stores Data
LLMs are designed to store vast amounts of parameters—variables derived from their training models—that they use to infer new content. A notable aspect of LLMs is their ability to "compress" the data they were trained on. This data-handling characteristic makes LLMs powerful tools for generating responses based on the knowledge embedded within them.
However, this training data can later be “regurgitated” in answers provided to users, as the New York Times’ copyright lawsuit against OpenAI highlighted.
This relationship between LLMs, the data sets from which they interact, and the systems in which they are integrated shows that the models themselves contain sensitive training data, underscoring the importance of robust cybersecurity and data privacy controls. To make an AI system truly useful to the enterprise, it must be trained and fine-tuned using actual corporate data—hardware and software designs, customer relationship management (CRM) data, financial records, and the like. LLM model “weights,” which represent the programming of the LLM, must be guarded carefully against exfiltration and tampering.
AI’s Enterprise Risks
As with many technological advances, the strengths of LLMs also introduce new challenges that can put an organization’s operations and data at risk of malicious and accidental events if the proper controls are not in place.
Some of the most notable enterprise risks include:
- Data Interception: Sensitive data, ranging from medical records to customer information, can be intercepted by leveraging AI technologies, posing a direct threat to individual privacy and organizational integrity.
- Poisoning Attacks: Attackers may manipulate data to cause AI tools to malfunction, potentially altering model results to produce malicious outcomes or introduce bias, undermining trust in AI-driven decisions and services.
- Exfiltration: An attacker who can get a copy of an AI model’s weights may be able to effectively copy the AI and query it for malign purposes.
As AI deployments grow and expand, the number of users who need to access the AI system grows and the location of these deployments expands. This AI sprawl can become more acute over time, making it difficult for cybersecurity teams to keep up with the exponential growth of the attack surface.
What You Can Do to Enhance Protection
Fortunately, there are some best practices and tools that organizations can use to balance the risks while maximizing the benefits of AI and LLMs:
Robust AI Auditing
As with any risk management program, the first step is to create and maintain a comprehensive inventory of all AI systems currently in use and subject them to regular audits. These audits should encompass thorough penetration testing and vulnerability assessments to pinpoint weaknesses within the AI infrastructure. By identifying these issues early on, organizations can promptly apply necessary patches or upgrades, thereby fortifying their defenses against cyber threats and ensuring security and privacy remain intact.
Prevent Unauthorized Access
Organizations often use AI and LLMs for a wide range of applications. This agility needs to be matched by a security model capable of protecting access without slowing down operations.
That’s where adopting a Zero Trust (ZT) framework—in combination with a strong access management program—becomes a cornerstone strategy. This security policy mandates that no entity within or outside the organization is trusted by default, emphasizing strict access control to who (or what) can access other network assets.
Such measures, when integrated with advanced, software-defined ZT platforms like Zentera, provide secure access and management solutions able to offer a layered defense against unauthorized access and prevent data leaks, such as model exfiltration. Deploying as an overlay, these solutions effortlessly expand security along with the AI deployment, allowing it to scale without overburdening IT and security teams.
Promote Responsible Use
Finally, in addition to technical controls and formal AI audits, organizations can utilize proven best practices:
- Educate Users: Educate users about how and when to use AI, its limitations, and security standards to prevent misuse of AI technologies.
- Develop Guidelines: Create comprehensive user guidelines to ensure consistent and ethical application of AI across your organization.
- Limit Personal Information Sharing: Advise users to limit the amount of proprietary, private, or personal information shared with AI systems to reduce compliance risks.
Bringing It All Together
The convergence of AI and cybersecurity presents both exciting opportunities and seemingly daunting challenges.
By understanding how AI (particularly LLMs) operates and stores data, organizations can better prepare themselves against cyber threats and implement robust, agile defense measures like Zero Trust to safeguard against AI-driven cybersecurity risks.
Want to see how your organization can harness the power of Zero Trust to navigate the complexities of AI and cybersecurity? Then take a moment to schedule a free consultation with a Zentera expert: